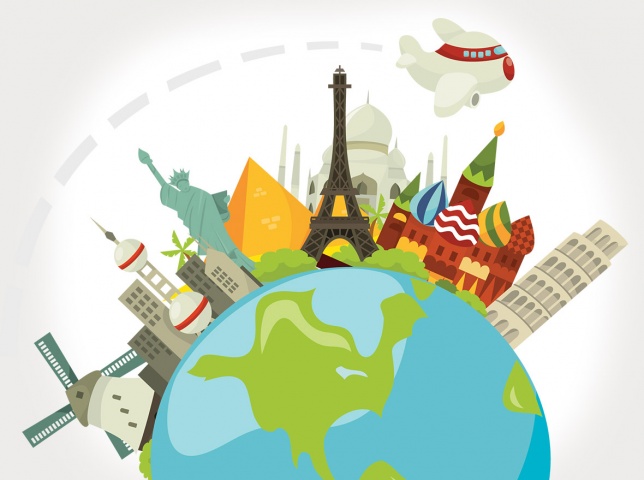
Matkakertomukset 2025
Vuoden 2025 matkakertomukset.
Koneoppimismenetelmät riskihenkivakuutusten raukeamisen ennustamisessa |
![]() |
|
In this work, the aim is to study selected machine learning methods and apply them in practice to a selected actuarial problem, namely the prediction of life insurance lapse risk. The selected machine learning methods were gradient boosting and neural networks.
First, a theoretical background for machine learning and the selected methods is presented. Second, the determinants of life insurance lapse risk and previous research on the topic are discussed. Finally, the process for applying the selected machine learning methods to life insurance lapse prediction is described and the modeling results are presented.
The results imply that the prediction of life insurance lapse can be improved by using machine learning. Both of the selected machine learning methods improved the lapse prediction results compared to more traditional statistical methods. Gradient boosting (XGBoost) performed slightly better than the neural network model and, especially taking into account the time efficiency of the fitting process and interpretability of the model, the XGBoost model was concluded to be better suited for lapse prediction.
The usefulness of the machine learning models in lapse prediction was also studied by using an economic metric for the lapse management. The result in terms of the economic metric was improved when the model was fit based on regression for the economic gain instead of using a pure classification. The actual economic gain, however, depends on how the parameters for the economic model are determined. Depending on the parameter selection, the resulting economic gain could remain limited or be quite significant. Therefore, the benefits of the lapse prediction depend on how efficient the insurance company is in their lapse management and on the overall profitability of the insurance product.
All in all, the machine learning methods are concluded to be useful in lapse prediction as well as other actuarial applications and their significance is also likely to increase in the future.
Vuoden 2025 matkakertomukset.
Olli Poutanen
Vuoden 2024 matkakertomukset.
Simo Poranen
Vesa Piilola
Petter Meyer
Tuula Stenfors
Iiro Marttila
Leo Viherä
Petri Hokkanen
Mikko Pelto
Blogi - Esko Kivisaari
Vuoden 2023 matkakertomukset.
Sami Hårdh
Teija Sonni-Kautto
Katariina Vaviolahti
Vuoden 2022 matkakertomukset.
Pekka Haapala
Roman Goebel
Blogi - Esko Kivisaari
Blogi - Janne Vieri
Tutkimuksessa tarkastellaan biologista ikää. Kronologinen ikä on yksilön (tavallinen) ikä, kun taas biologinen ikä on se ikä, jonka ikäiseltä yksilö vaikuttaa.
Blogi - Janne Vieri
Vuoden 2021 matkakertomukset.
Blogi - Esko Kivisaari
Eveliina Rikberg
Jaakko Aho
Sääntelytyöryhmä on laatinut keskustelupaperin yhteistakuujärjestelmään liittyvien Eurooppalaisten kaavailujen ja mahdollisen harmonisoinnin osalta sekä erityisesti käynyt asiaa Suomen näkökulmasta.
Panu Pursiainen
Mari Leppänen
Marko Lehtovirta
Minna Lehmuskero
Vuoden 2020 matkakertomukset
Tuija Nopola
Vuoden 2019 matkakertomukset
Aarno Ahteensivun, Lasse Koskisen, Jarna Kulmalan ja Pauliina Havakan toimittamassa kirjassa esitellään monipuolisesti erilaisia riskeihin ja riskienhallintaan liittyviä teemoja.
Hanna Mäkinen
Vuoden 2018 matkakertomukset
Ville Lilja
Kari Lahti
Henrik Jämsä
As populations age over the next several decades, the demand for long-term care (LTC) services (assisting individuals with their activities of daily life) will increase dramatically and is likely to reach crisis levels in many countries.
Tuomas Keski-Kuha
Eki Vikman
Anne Setämaa-Kärkkäinen
Vuoden 2017 matkakertomukset
Jukka Johansson
Teemu Hänninen
Jani Pajukangas
Jesse Kolponlahti
A guide for practitioners interested in understanding this important emerging field, "Stochastic Modeling" presents the mathematical and statistical framework necessary to develop stochastic models in any setting (insurance or otherwise).
The time value of money is significant to economics and in turn to the current value of projected cash flows. Recent decades have seen a growth of knowledge and available information in the areas of finance and capital markets.
Ismo Risku
Tapio Isolankila
Vuoden 2016 matkakertomukset
Hanna Eskola
Antti Huotari
Jenni Pokkinen
Vuoden 2015 matkakertomukset: IAA Zürich, IAA vuosikokous Vancouver, AAE vuosikokous, SFPC-raportti.
Rantala Jukka 2015: Eläkejärjestelmää on syytä arvioida monipuolisesti
Toni Blomster
Olli Punkkinen
Vesa Kuikka
Erkka Jalonen
Tiina Seppänen
Teija Talvensaari
Mika Sirviö
Vuoden 2014 matkakertomukset.
Satu Korhonen
Martti Pesonen, Pentti Soininen ja Tapani Tuominen 2014: Henkivakuutusmatematiikka
Henkivakuutusmatematiikka-kirjasta on ilmestynyt uusi painos elokuussa 2014. Edeltävästä painoksesta on ehtinyt vierähtää jonkinmoinen tovi...
Rantala, Jukka; Kivisaari, Esko 2014: Vakuutusoppi
Jokaisen finanssialalla työskentelevän on tärkeää ymmärtää vakuuttamisen lainalaisuuksia ja eri vakuutuslajien pääpiirteitä. Vakuutusoppi mahdollistaa kokonaiskuvan s...
Åsa Backlund
Mathias Brunssberg
Tarja Sirén
Annina Pietinalho
Tuomas Marttila
Matias Leppisaari
Vuoden 2013 matkakertomukset.
Tuomas Hakkarainen
Teppo Rakkolainen
Anssi Puranen
Vuoden 2012 matkakertomukset.
Matti Huovila
M Karhunen
Antti Pulkkinen
Olof Eriksson
Riitta Aitio
Esko Prokkola
Uolevi Mannonen
Esa Säyrinen
Heikki Peltola
Markku Vesterinen
Nils-Erik Felixsson
Olavi Tenni
Erkki Nokelainen
Markku Karhunen
Yrjö Tiira
Leo Wistbacka
Mikko Päivinen
Kari Puustinen
Petri Vilska
Sari Martikainen
Rantala Jukka 2011: Kommentti Sixten Korkmanin artikkeliin "Kenelle valta päättää työeläkkeistä?"
Vuoden 2011 matkakertomukset.
D'Ambrogi-Ola, Barbara 2011
Rantala Jukka 2011: Virastosta tietotaloksi
Matti Tienari
Jussila Antti 2010
Vuoden 2010 matkakertomukset.
Tienari Matti 2010
Lauri Saraste
Antti Auranen
Kolehmainen Piia 2009
Vuoden 2009 matkakertomukset.
Tony Airio
Laakso Sanna 2009
Sami Tähtinen
Pentikäinen T, Rantala J 2009
Vakuutusalan perusoppikirja: historian, käsitteiden ym sselityksiä, vakuutuslajien lyhyt esittely, sekä sosiaali- että yksityisvakuutus.
Ropponen Sari 2008
Lauri Ojala
L Ojala
Anu Imppola
Vuoden 2008 matkakertomukset.
Samu Anttila
Janne Sorainen
Pekka Sihvonen
Vuoden 2007 matkakertomukset.
Mikko Kuusela
Tarja Taipalus
Rantala Jukka 2007: The Actuary
Niittuinperä Jari 2007
Sauli Karonen
Markku Kärnä
Vuoden 2006 matkakertomukset.
Rantala Jukka 2006: ASTIN Bulletin 2006 The Actuary August 2006
Rantala Jukka 2006: Festschrift for Tarmo Pukkila on his 60th birthday
Mari Alho
M Alho
Kauppi Mira 2005
SHV-nro 80
Jarkko Pajunen
Vuoden 2005 matkakertomukset.
Janne Kaippio
Tiina Nurmi
Tony Silander
Rantala Jukka 2005: Nordisk Försäkringstidskrift
Carin Lindqvist-Virtanen
Heikki Arminen
Åsa Ceder
Markku Miettinen
Vuoden 2004 matkakertomukset.
Jorma Kinnunen
Janne Pesonen
Mika Mäkinen
Mikko Heikkilä
Eero Heinilä
Teemu Jokela
Satu Kilponen
Vuoden 2003 matkakertomukset.
Rantala Jukka 2003: Focus, SCOR Group
Kimmo Karppinen
Vuoden 2002 matkakertomukset.
Mari Savela
Korpela Timo, Ranne Antero 2002: Eläkevakuutus
Kirjoituksessa esitetään keskimääräisen eläkkeelle siirtymisen iän laskemiseksi menetelmä, joka perustuu tilaluokkien välisiin siirtymätodennäköisyyksiin.
Rantala Jukka 2002: Selvitysmiehen raportti, Sosiaali- ja terveysministeriö, Työryhmämuistioita
Merja Mäkitalo
Vuoden 2001 matkakertomukset.
Merja Lassila
Sokka Antti 2000: Savon Sanomat
Pentikäinen Teivo 2000: Talouselämä
Teivo Pentikäinen palaa kysymykseen, voivatko ulkoatuodut VIP-henkilöt koskaan onnistua vakuutusjohtajina.
Pentikäinen Teivo 2000: Kauppalehti
Yksi useista artikkeleista, joissa kritisoitiin omistajalähtöisyyttä, Iiro Viinasta, Pirkko Alitaloa sekä If-hanketta.
Harri Kuosmanen
Vuoden 2000 matkakertomukset.
Päivi Ojala
Rantala Jukka 2000: Giornale dell\'institute italiano degli attuari
Heinonen Erja, Nokelainen Erkki, Ranne Antero, Tuomikoski Jaakko, Tuomisto Päivi 2000: Työeläke
Kirjoituksessa esitellään Ilmarisessa tehty arvio kokonaiseläkekustannuksista suhteessa palkkoihin 15 Euroopan maassa. Eläkk...
Pentikäinen T. - Virtanen Matti 2000
Yksi Teivo Pentikäisen lukuisista lausunnoista ja muistioista Kansa-yhtiöiden konkurssiprosessin kuluessa.
Ranne Antero 2000
Ranne Antero, Kivisaari Esko, Mannonen Hillevi 2000
Rantala Jukka 2000: Giornale dell\'istituto Iialiano degli attuari
Vuoden 1999 matkakertomukset.
Pasi Laaksonen
Pentikäinen Teivo 1999: Nykypäivä
Keskustelua siitä, tarvitaanko vielä sinivalkoisuutta vai korvaako globaalisuus sen kokonaan.
Tuomikoski Jaakko 1999: Vakuutuslääketiede
Artikkeli on yleisesitys vakuutustoiminnan yleisistä periaatteista ja selostaa vakuutustoiminnan käsitteitä (Vakuutus, riski, vakuutusten luokittelu, vakuutuksenantaja, keskinäine...
Vesikansa Jyrki 1999: Kauppalehden Optio
Newco oli If-yhtiön väliaikainen nimi.
Tuomikoski Jaakko 1999
Kirjassa esitellään lakisääteisen työeläkevakuutuksen vakuutustekniikkaa sellaisena kuin se oli kirjan ilmestymisvuonna. Laskuperusteiden tärkeimpien periaateratkaisujen ja olennaisimpien kaavojen o...
Pentikäinen Teivo 1999: British Actuarial Journal
C.D. Daykinin ja D. Lewisin A Crisis of Longer Life -raportin keskusteluosassa Teivo Pentikäinen selostaa ikäongelman näkökohtia Suomessa ja sitä, miten niistä uskotaan se...
Pentikäinen Teivo 1999
Kriittistä keskustelua ostoeläkkeistä ja erityisesti niiden huonosti harkitusta verotuksesta.
Ranne Antero 1999: Vector
Rantala Jukka 1999: Giornale dell\'istituto Iialiano degli attuari
Lindell Christina 1999: Eläketurvakeskuksen raportteja
Kuolevuuden trendinomainen aleneminen ja elinajan vastaava piteneminen ovat jatkuneet pitkään. Erityisesti ikääntyneiden kuolevuus on pienentynyt viimeisten 50 vuoden ...
Jaakko Tuomikoski, Janne Sorainen ja Satu Kilponen (2007)
Käsikirjassa esitellään työeläkejärjestelmän rahoitusperiaatteet ja laskuperustemalli sekä malliin liittyvät vakuutusmatemaattiset kaavat ja vakiot.
Pentikäinen Teivo 1998: Kauppalehti
Teivo Pentikäinen ihmettelee sellaista Kauppalehdessä julkaistua käsitystä, että vakuutusvalvojan ei tarvitse olla edes vakuutustoiminnan asiantuntija.
Pentikäinen Teivo 1998: Kauppalehti
T. Pentikäinen kritisoi ns. ostoeläkkeiden markkinointiin liittyviä seikkoja.
Mikko Karpoja
Pentikäinen Teivo 1998: Taloussanomat
Teivo Pentikäinen toteaa Iiro Viinasen levittävän vääriä tietoja siitä, että eläkkeiden rahoitus ei olisi kunnossa.
Jukka-Pekka Kurki
Ahonen Antero, Hietaniemi Marjukka, Jokinen Pia, Kivisaari Esko, Laitinen-Kuikka Sini, Lindell Christina, Lundqvist Bo, Neuvonen Kati, Nokelainen Erkki, Saarnio Leena, Turunen Juhani, Voivalin Matti 1998
Kirjassa suomalaiset asi...
Ainassaari Katja, Kallio Markku, Ranne Antero 1998
Korpela Timo - Pentikäinen Teivo 1998: Transactions of the 26th International Congress of Actuaries
Keskustelua väestön ikääntymisestä johtuvista ongelmista erityisesti korostaen sitä, että eläkeläistymisen myöhentäm...
Pentikäinen Teivo 1998
Keskustelua lamasta liittyen mm. Kianderin ja Vartian kirjaan Suuri lama. Laman syitä ja seurauksia, vaikutuksia vakuutusalalla nyt ja ehkä tulevaisuudessa.
Pentikäinen Teivo 1998: The Actuary
Teivo Pentikäinen kertoo riskiteorian soveltamisesta Suomessa.
Ranne Antero 1998
Tuula Lempiäinen
Pentikäinen Teivo 1997: Helsingin Sanomat
Kritiikkikirjoitus (yksi monista) Björn Wahlroosin ehdotusten johdosta. Hän olisi kirjoittanut eläkelait kokonaan uusiksi ja yhdistänyt rahoitus- ja vakuutustarkastukset.
Pentikäinen Teivo 1997: ABaCuse
Esitelmä riskiteorian sovellutuksista Ruotsin aktuaariyhdistyksessä.
Pentikäinen Teivo 1997: Kauppalehti
Kritiikkiä muotiin pääsemässä ollutta äärimmäisyyksiin vietyä omistajalähtöistä johtamiskulttuuria vastaan, joka on vaarassa unohtaa vakuutusasiakkaat.
Näräkkä Aimo - Pentikäinen Teivo 1997: Helsingin Sanomat
Protestoitiin Suomi-yhtiön osakeyhtiöksi muuttamista vastaan. Tästä alkoi yli kolme vuotta kestänyt prosessi, joka lopulta kaatoi Iiro Viinasen ja Pirkko Alitalon...
Kai Niemi
Pentikäinen Teivo 1997: Vakuutussanomat
Debattikirjoitus vakuutusalalle leviämässä ollutta omistajalähtöisyyttä ja globaalisuutta vastaan.
Pentikäinen Teivo 1997: Talous & yhteiskunta
Keskustelua ikääntymisongelmasta.
Pentikäinen Teivo 1997: Eläkepolitiikka 2000-luvulle
Työeläkejärjestelmän synty, tausta, periaatteet ja tulevaisuuden näkymät.
Pasi Mustonen
Raoul Berglund
Daykin Chris - Pentikäinen Teivo - Pesonen Martti 1996: The Actuary
Jatkettua väittelyä riskiteorian teoreettisen ja käytännön (practical) koulukunnan välillä. Daykin, Pentikäinen ja Pesonen katsovat, että on täysin k...
Pentikäinen - Koivusalo - Kammonen - Lämsä 1996
Selostus työeläkkeistä, mukaanlukien myös valtion ja kuntien järjestelmät. Jatkoa Teivo Pentikäisen alkujaan yksin laatimalle kirjalle.
Pentikäinen Teivo 1996
Synonyymisanakirja, jossa vakuutusalan keskeisiä käsiteitä on määritelty ja ryhmitelty luonnollisiin yhteyksiinsä ja varustettu käytön esimerkein. Peter Johnson avusti kirjan toimittamisessa.
Ranne Antero 1995
Kirjoituksessa kehitetään sijoitustuottojen simulointimalli, joka liitetään osaksi TEL-yhtiötä kokonaisuutena kuvaavaa mallia. Tätä käytetään arvioimaan toimintapääoman tarpeen riippuvuutta yhtiön...
Pentikäinen Teivo 1995: Kauppalehti
Keskustelua siitä, tulisiko vakuutusalalla olla yhteisvastuujärjestelmä, josta korvattaisiin avoimeksi jääneet vahingot vakuutusyhtiön joutuessa konkurssiin.
Pentikäinen T, Rantala J 1995
Vakuutusalan perusoppikirja: historian, käsitteiden ym. selityksiä, vakuutuslajien lyhyt esittely, sekä sosiaali- että yksityisvakuutus.
Tuomikoski Jaakko 1995
Ranne Antero 1995
Pertti Pulkkinen
Marika Vilska
Pentikäinen Teivo 1994: Helsingin Sanomat
Yksi useista puheenvuoroista siitä, olisiko vakuutuskorvausten turvaksi tarpeen aikaansaada vakuutuslaitosten yhteistakuu. Idea lopulta toteutui kuitenkin vain lakisääteisten vakuutu...
Ahjos Timo 1994
VALIKO-koulutustilaisuudet (Vakuutusalan liikkeenjohdon jatkokoulutus) 1993-94.
Koulutustilaisuuden tueksi laadittu moniste, jonka liitteenä Talouselämä-lehdessä julkaistu 2-sivuinen artikkeli \"Kuka vakuut...
Daykin C.D. - Pentikäinen T. - Pesonen M. 1994
Uusittu riskiteorian oppikirja. Kirja perustuu brittien ja suomalaisten lukuisiin aikaisempiin tutkimuksiin.
Pentikäinen T. 1994
Kriittistä debattia Jaakko Lassilan vakuutusalalle tuomaa markkinahenkisyyttä vastaan (markka ja ääni -periaate) ja Lassilan vakuutusuran arviointia.
Pirkko Welin-Siikaluoma
Esko Kivisaari
Kalervo Koistinen
Saajoranta Atso, Saarinen Kaija-Riitta, Makkonen Pekka 1993
Artikkeli käsittelee suomalaisen työeläkevakuutusyhtiön vastuuvelan ja sen katteen keston mukaan segmentoitua analyysia ja siihen liittyvää mallia.
Hely Salomaa
Pentti Soininen
Jarmo Jacobsson
Risto Heimo
Juha-Pekka Halmeenmäki
Hillevi Mannonen
Heikki Bonsdorff
Pentikäinen T. - Rantala J. 1992: ASTIN Bulletin
Ensin simuloidaan vahinkomenojen virtaa ja sitten tietokone pannaan leikkimään aktuaaria, joka tästä virrasta laskee korvausvastuut. Käyttämällä eri menetelmiä saadaan k...
Pentikäinen Teivo 1992: Suomen Kuvalehti
Keskustelua finanssitavarataloista ja niihin liittyvistä riskeistä.
Ranne Antero 1992
Rantala J. 1992: Nordisk Försäkringstidsskrift
Pentti Tervola
Leena Väänänen
Anni Sajo
Pentikäinen Teivo 1991
Juhlaesitelmä Ilmarisen hallintoneuvoston kokousta varten 18.11.1991
Rantala J. 1991: Kansantaloudellinen Aikakauskirja
Rantala J. 1991: Managing the insolvency risk of insurance companies
Rantala J. 1991: Aktuaaripäivän 15.1.1990 esitelmät
Yrjö Romppainen
Jari Sokka
Pentikäinen Teivo 1990
Vastuunkantokyvyn arviointi riskiteorian keinoin.
Erkki Kautto
Irmeli Saari
Pentikäinen - Bonsdorff - Pesonen M. - Rantala - Ruohonen 1989
Työryhmä \"FIM-Group\" laati laajan stokastis-dynaamisen analysointimallin vakuutusliikkeestä. Joukko tärkeitä sovellutuksia otettiin mukaan. Tämä kirja esit...
Rantala J. 1989: ASTIN Bulletin
Simo Sarvamaa
Erkki Immonen
Onerva Savolainen
Pertti Savijoki
Ahtokari Reijo (Pentikäinen T.) 1988
Teivo Pentikäisen muisteluita, jotka kokosi kirjaksi Reijo Ahtokari. Käsitellään sekä vakuutustoiminnan että eläkejärjestelmien kehitystä sodan jälkeisinä vuosikymmeninä.
Pentikäinen T. 1988: SIAM Review
Vastaus tri Nashille, joka oli arvioinut Risk Theory -teoksen (Beard, Pentikäinen, E. Pesonen) uuden, kolmannen painoksen huonosti riskianalyytikoiden tarkoitukseen sopivaksi. Teivo Pentikäine...
Tuomikoski Jaakko 1988
Pentikäinen Teivo 1988
Ikääntymisongelma eläkejärjestelmien ja koko yhteiskunnankin kannalta. Syitä, seurauksia ja toimenpiteitä. Avustajina mm. gerontologian professorit Hervonen ja Ilmarinen.
Pentikäinen Teivo 1988: Handwörterbuck der Versicherung HdV
Lyhyt selostus solvenssista suureen saksalaiseen vakuutustietosanakirjaan.
Ranne Antero, Ryynänen Markku 1988
Rantala J. 1988: Transactions of the 23rd International Congress of Actuaries
Rantala J. 1988: Transactions of the 23rd International Congress of Actuaries
Rantala J. - Hietikko H. 1988: European Journal of Operational Research
Ari Ikonen
Pentikäinen Teivo 1987: ASTIN Bulletin
Vahinkomenon jakauman tavallisimpia approksimaatioita (NP-, Wilson-Hilferty - ja Haldane-menetelmät) on verrattu tarkkoihin tuloksiin, jotka on laskettu rekursiokaavalla. Tulosten avulla ...
Harri Nyrhinen
Tuomikoski Jaakko 1986
SHV-työ.
Pentikäinen Teivo 1986: Kauppalehti
Esimerkki lukuisista puheenvuoroista keskustelussa eläkepommista.
Pertti Näyskä
Pentikäinen T. 1986: Insurance: Mathematics and Economics
Kommentti Taylorin kilpailuolosuhteita koskevalle kirjoitukselle. Vakuutuslaitosten välisen kilpailun mallittamista, syklit, vakuutusten karkaaminen jne.
Pentikäinen T. - Rantala J. 1986: ASTIN Bulletin
Korvausvarausten epätarkkuudesta johtuva ns. run-off risk esitetään omaksi riskin lajikseen liitettäväksi riskiteorian standardimalliin.
Pentikäinen Teivo 1986: Työeläke
Vakuutuspoliittinen puheenvuoro: Lakisääteisiä vakuutuksia hoitavat vakuutusyhtiöt ovat vastuussa myös siitä, että laki on ajantasalla, eikä siihen sisälly epäkohtia. Velvollisuus on...
Pentikäinen Teivo 1986
Vakuutusyhdistyksen 75-vuotispäivän juhlaesitelmä 15.4.1986. Sisältää katsauksen Suomen vakuutustoiminnasta vuosilta 1945-1980.
Rantala J. 1986: ASTIN Bulletin
Rantala J. 1986: Suomen tilastoseuran vuosikirja 1985
Heikki Ranta
Olli Pusa
Hopia Ara - Hedenström Kaius 1985: Vakuutussanomat
Teivo Pentikäinen voimakkaasti korostaa sitä, että vakuutusalan johtotehtäviin kelpaavat vain alaa osaavat henkilöt, eivät erilaiset ns. VIP-ihmiset, joiden värväämine...
Pentikäinen T. 1985: Giro Bulletin
Giro Bulletin oli myöhemmän The Actuary -lehden edeltäjä Isossa-Britanniassa. Tässä artikkelissa mm. keskustellaan siitä, miksi riskiteoriaan Isossa-Britanniassa ja muuallakin suhtaudut...
Pentikäinen Teivo 1985
Esitelmä osuustoiminnallisten vakuutusyhtiöiden seminaarissa.
Pentikäinen Teivo 1985: Työeläke
Julkisella sektorilla eläkeikä oli aiemmin ammattikohtaisesti erilainen. Seurauksena oli jatkuvia paineita, jopa lakkoja, kunkin ammattiryhmän eläkeiän alentamiseksi. Kuntasektorilla tila...
Rantala J. 1985: Nordisk Försäkringstidskrift
Rantala J. 1985: The Review
Reijo Sihvonen
Jari Saine
Lasse Heiniö
Beard R. E. - Pentikäinen T. - Pesonen E. 1984
Käytäntöön orientoitunut riskiteoria, brittiläisen ja suomalaisen senaikaisen osaamisen kokooma. Siemenä oli Suomessa monisteena julkaistu Riskiteoria (ks. Pentikäinen, 1955...
Rantala J. 1984: Transactions of the 22nd International Congress of Actuaries
Rantala J. 1984
Raimo Voutilainen
M Ruohonen
Matti Ruohonen
Pentikäinen T. 1983: European Journal of Operational Research
Selostus vakuutuslaitoksen riskiteoreettisesta mallittamisesta.
Pentikäinen Teivo 1983
Keskustelua Englannin solvenssiryhmän kanssa. Näin pohjustettiin yhteistä riskiteorian kirjaa.
Rantala J. 1983: Festschrift for Eino Haikala on his seventeeth birthday
H Bonsdorff
Pentikäinen T. 1982: ReActions
Osallistuminen brittien keskusteluun aktuaarien tehtävistä ja tarpeellisuudesta.
Pentikäinen Teivo 1982
Uusien pöytätietokoneiden kokokapasiteettiä käytettiin ensimmäisen kerran vakuutusliikkeen stokastiseen mallittamiseen. Tämä oli alkuna vastaavalle laajalle käytännölle, mm. Monte Carlo -idean m...
Rantala J. 1982
Eero Gauffin
Pentti Koskinen
Seppo Koskinen
Juhani Heiskanen
Pentikäinen T. 1981
Suomessa ehdotettu ja sittemmin toteutettu joustavien eläkeikien järjestelmä selostettuna kansainväliselle lukijakunnalle.
Pentikäinen T. - Rantala J. 1981: ASTIN Bulletin
Riskinkantokyvyn arviointi stokastisen mallin avulla.
Markku Paakkanen
Pentikäinen T. 1980: Vakuutussanomat
Keskustelua vakuutuksen määrittelemisyrityksistä.
Pentikäinen T. 1980: Transactions of the International Congress of Actuaries
Ajanmukaistettu ja uusittu selostus riskiteoreettisesta mallittamisesta.
Pentikäinen T. 1980
Vahinkomenon likimääräisiä laskentatapoja.
Pentikäinen Teivo 1980: Kodin Kuvalehti
Keskustelua eläkeikäjärjestelmästä. Tässä artikkelissa ennakoitiin sittemmin komiteassa toteutettua järjestelmää, joka salli tietyissä rajoissa yksilöllisen eläkkeelle siirty...
Pentikäinen Teivo 1980: Gioruale dell\' Istituto Italiano degli Attuari
Lähinnä italialaisia aktuaareja varten kirjoitettu selostus Suomen solvenssijärjestelmästä.
Pentikäinen Teivo 1980: The Journal of Risk and Insurance
Selostus Euroopassa kehitetyn riskiteorian sovellutuksista USA:n yliopistojen riskiteoriaseminaarille.
Alpo Mustonen
Pentikäinen T. 1979: ASTIN Bulletin
Keskustelua siitä, miten riskiteorian avulla voidaan arvioida kilpailutilanteeseen liittyviä riskejä ja miten alas esim. vakuutusmaksuja on terveellistä alentaa.
Pentikäinen Teivo 1979: Sosiaalinen Aikakauskirja
Perusteellinen selvitys työ- ja muiden eläkejärjestelmien ideoista, tilasta ja tulevaisuudesta.
Pentikäinen T. 1978: Scandinavian Actuarial Journal
Vakuutusliikkeen mallittamisen ensimmäisiä askeleita riskiteoriaa hyväksikäyttäen.
Pentikäinen Teivo 1978
Debattia eläkejärjestelmien kustannuksista. Tiivistelmä on julkaistu myöhemmin Sosiaalisessa Aikakauskirjassa.
Pentikäinen Teivo 1978: Suomen Kuvalehti
Puheenvuoro eläkekustannusdebatissa.
Pentikäinen Teivo 1978: Suomen Kuvalehti
Puheenvuoro eläkekustannusdebatissa.
Seppo Eskelinen
Pentikäinen T. 1977: ASTIN Bulletin
Korvausmenon approksimatiivisen laskennan menetelmiä ja arviointia.
Pentikäinen Teivo 1977: Sosiaaliturva
Eläkejärjestelmät vakiinnuttaneen ns. historiallisen kompromissin perustelut ja vaikutukset.
Pentikäinen Teivo 1977
Kritiikkiä sellaisia käsityksiä vastaan, että vapaaehtoiset yksilölliset eläkejärjestelyt pystyisivät täyttämään minkään valtakunnan todellista eläketurvantarvetta.
Pentikäinen Teivo 1977: Sosiaalinen Aikakauskirja
Puheenvuoro debatissa siitä, ovatko työnantajien työeläke- ym. sosiaalikustannukset liian korkeat.
Pentikäinen Teivo 1977: Sosiaalinen Aikakauskirja
Työeläkejärjestelmän ideoitten esittelyä.
Jorma Leinonen
Hannu Parviainen
Yrjö Turtiainen
Pentikäinen T. 1976: Transactions of the International Congress of Actuaries
Tietokoneiden mahdollistama simulointi vakuutusyhtiöiden tilan ja tulevaisuuden selvittämiseksi.
Saajoranta Atso, Saarinen Kaija-Riitta, Makkonen Pekka 1976: Forecasting in Pension Insurance Field
Kirjasessa on kuvattu sellaisten ennustemallien periaatteet, joita on käytetty sekä TEL- että YEL-vakuutusliikkeen 2-20 vuode...
Pentikäinen Teivo 1976
Dynaamisen ohjelmoinnin ja tietokoneiden simulointikäytännön sovellutusten selostus.
Pentikäinen Teivo 1976: Sosiaalinen Aikakauskirja
Puheenvuoro debatissa siitä, onko Suomessa eläketurva kehittynyt liian kalliiksi vaarantaen mm. suomalaisten yritysten kilpailukyvyn. Tällaiset käsitykset torjuttiin, mutta ...
Tuomas Hirvonen
Pentikäinen T. 1975: Scandinavian Actuarial Journal
Ensimmäisiä yrityksiä käyttää suuresti kehittyneitä tietokoneita vakuutusyhtiöiden solvenssin ja tulevaisuuden arvioitiin.
Pentikäinen Teivo 1972: Vakuutussanomat
Aikaisemmin oli tapana laskea vakuutustoiminnan kannattavuutta vertaamalla vakuutusmaksuja korvaus- ym. kuluihin. Tässä artikkelissa katsottiin, että kannettavuuslaskelmiin ja erityise...
Pentikäinen Teivo 1972: Insurance in Finland
Suomen sosiaalivakuutuksen erityispiirteiden esittelyä kansainväliselle lukijakunnalle, erityisesti työeläkkeiden ja tapaturmavakuutuksen hajasijoitettu organisaatio.
Pentikäinen Teivo 1971
Lyhyt selostus sosiaalivakuutuksen historiasta, käsitteistä ja senhetkisestä tilasta. Tämä teksti on myöhemmin siirretty osaksi Vakuutusoppia.
Pentikäinen Teivo 1970: Insurance in Finland
Suomen tasoitusvastuun selostus kansainväliselle lukijakunnalle.
Markku Lehtinen
Antti Törrönen
Taisto Paatsila
Pentikäinen Teivo 1968: Transactions of the International Congress of Actuaries
Selostus suomalaisen indeksisidonnan saavutuksista. Erilaisia teoreettisia ja käytännön ratkaisumuotoja selostetaan ja luokitellaan. Suuri innov...
Pentikäinen T. 1967: ASTIN Bulletin
Selostus kansainväliselle lukijakunnalle Suomen silloin jo hyvin kehittyneestä vakavaraisuusjärjestelmästä.
Pentikäinen T. 1967: Helsingin Lääkärilehti
Yrittäjäasemassa olevien henkilöiden eläkejärjestelmien kehittämisideoita.
Pentikäinen Teivo 1967: Huoltaja
Alusti kysymystä perhe-eläkkeen etujen kehittämiseksi siten, että molemmat sukupuolet olisivat tasavertaisessa asemassa ja eläkkeen saanti ja määrä olisi porrastettu muuta kautta saatavi...
Pentikäinen Teivo 1967
Matemaattisten kaavojen kokoelma lähinnä käsittäen oppikoulumatematiikan sekä korkeakoulujen ensimmäisen vuoden oppimääriä.
1. painos 1947
Pentikäinen Teivo 1966: Vakuutussanomat
Puheenvuoro keskusteluun siitä, pitäisikö mm. vakuutus määritellä \"tieteellisesti\".
Pentikäinen Teivo 1965: Vakuutussanomat
Indeksiehdon kehittäminen oli suomalaisten aktuaarien suursaavutuksia sodan jälkeisissä inflatorisissa sekavissa oloissa. Tässä Vakuutussanomien artikkelissa kerrotaan menestystarina...
Pentikäinen Teivo 1963: Yhteiskuntatieteiden käsikirja. 1. osa
Eläkkeiden perusteista kansantajuinen selostus
Pentikäinen Teivo 1962: Uusi Suomi
Yleisselostus työntekijäin eläkelaista.
Pentikäinen T. 1962: Liiketyönantajain Keskusliitto Liiketyönantajain Keskusliiton julkaisuja
Kun lakisääteinen työeläkejärjestelmä oli säädetty vuonna 1961, julkaistiin sikermä vihkosia ja artikkeleja, joissa asiaa ...
Pentikäinen Teivo 1962: ASTIN Bulletin
Riskiteorian sovellutuksena käsiteltiin autovakuutuksen vastuuvelan määritystä.
Pentikäinen Teivo 1962: ASTIN Bulletin
Riskiteorian sovellutuksena keskustellaan jälleenvakuutuksen ja sen omavastuiden valinnasta.
Pentikäinen Teivo 1962: Liiketalous
Selostus työntekijäin eläkelaista
Pentikäinen Teivo 1962: Liiketaloudellinen Aikakauskirja
Selostusta uudesta työeläkelainsäädännöstä.
Pentikäinen Teivo 1962: Insurance in Finland
Työeläkejärjestelmää selostetaan englannin kielellä.
Pentikäinen Teivo 1962
Yleisselostus työeläkejärjestelmän yksityiskohdista ja periaatteista. Tämä kirja yhdistettiin Työntekijän eläke -kirjaan.
Pentikäinen Teivo 1961: Nordisk Försäkringstidskrift
Keskustelua siitä, olisiko työkyvyttömyyden kohdatessa työkyvyttömyyseläkkeen asemesta suoritettava tehokasta kuntoutusta ja pyrittävä palauttamaan ao. henkilö tak...
Pentikäinen Teivo 1961: Oma maa: tietokirja Suomen kodeille
Lyhyt yleistajuinen selostus sosiaalivakuutuksen silloisesta tilasta.
Pentikäinen Teivo 1961: Vakuutussanomat
Provokatoorisesti tarjottiin kuntoutusta aina ensisijaisena toimenpiteenä ja työkyvyttömyyseläkkeen myöntäminen vasta toissijaisena. Artikkeli oli ajan hengen mukaisesti ylioptimist...
Pentikäinen Teivo 1961
Selostus työeläkkeistä, mukaanlukien myös valtion ja kuntien järjestelmät. Myöhemmin julkaistu uudistettuna nimellä Työeläke.Selostus työeläkkeistä, mukaanlukien myös valtion ja kuntien jär...
Pentikäinen Teivo 1959: Sähköurakoitsijalehti
Yleisselostus työntekijäin eläkelaista. Tämä on esimerkki lukuisista artikkeleista, joita julkaistiin eri lehdissä.
Pentikäinen T. 1957: Transactions of the International Congress of Actuaries
Suomen eläkesäätiöinstituution selostusta.
Pentikäinen Teivo 1957
Tällä monistetulla muistiolla oli erittäin suuri merkitys vakuutusalalle. Henkivakuutusyhtiöt toivoivat korotuksia vakuutusmaksuihin. Tässä muistiossa kuitenkin asetuttiin sille kannalle, että henk...
Pentikäinen Teivo 1957
Vakuutusalan perusoppikirja: historian, käsitteiden ym. selityksiä, vakuutuslajien lyhyt esittely, sekä sosiaali- että yksityisvakuutus. Käännetty ruotsiksi v. 1968 (Försäkringslära).
Pentikäinen T. 1954: Transactions of the International Congress of Actuaries
Selostus siitä, miten riskiteorian avulla voidaan määrittää jälleenvakuutuksen omavastuiden tasoa.
Pentikäinen Teivo 1954
Komiteatyö, jonka pohjalta perustettiin uusi eläkejärjestelyinstituutio eräänlaiseksi täysin turvallisen vakuutuksen ja vailla mitään vakuuksia toimivan eläkesääntökäytännön välimuodoksi.
Pentikäinen Teivo 1953: Nordisk Försäkringstidskrift
Suomen uutta vakuutusyhtiölakia esiteltiin pohjoismaisille lukijoille ruotsinkielisessä artikkelissa.
Pentikäinen T. 1952: Scandinavian Actuarial Journal
Suomen juuri käytäntöön otettua turvajärjestelmää selostetaan kansainväliselle lukijakunnalle. Osoitetaan myös, miten riskiteorian keinoin voidaan määrittää jäll...
Pentikäinen T. 1952
Vakuutusyhtiölakikomitean mietinnän (6/1952) liite, jossa perustellaan lakiin otettua turvajärjestelmää. Käytäntöön tulivat riskistä riippuvat pääomavaatimukset sekä riskisyklejä tasoittava tas...
Pentikäinen Teivo 1947: Scandinavian Actuarial Journal (Skandinavisk Aktuarietidskrift)
Tässä vaatimattomassa julkaisussa ensimmäisen kerran Suomessa pyrittiin soveltamaan riskiteoriaa käytännön ongelmaan.
Pentikäinen Teivo 1947
Väitöskirja. Tehtävä oli löytää kaikki ne funktiot, joilla on se ominaiuus, että arvo pisteessä x+y on jokin algebrallinen funktio A saman funktion arvoista pisteissä x ja y, siis f(x+y)=A[f(x),...
Pentikäinen Teivo 1946: Avustuskassojen Yhdistyksen kiertokirje 10/5.6.1946
Esitelmä Avustuskassojen Yhdistyksen vuosikokouksessa 5.5.1946
Suunnitelma riskihenkivakuutuksesta, jossa vakuutusmaksu on kiinteä (kuitenkin inde...